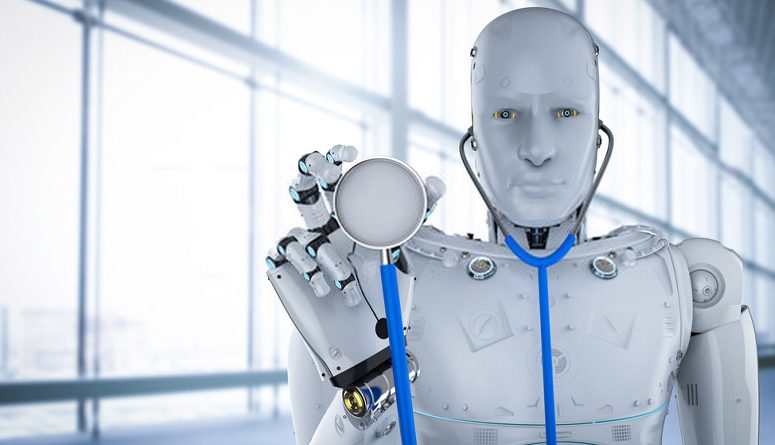
An artificial intelligence (AI) system was equivalent to or better than radiologists at reading mammograms for high-risk cancer tumors that required surgery, according to a 2017 study from the Massachusetts General Hospital and MIT. Google had previously demonstrated, as reported in the Journal of the American Medical Association, that computers can examine diabetic retinal images in the same way that ophthalmologists can. Recently, computer-controlled robots successfully conducted intestinal surgery on a pig. While the robot took longer than a human, the robot’s sutures were far superior—more exact and uniform, with fewer risks of breakage, leaking, or infection. AI proponents claim that the technology will result in better evidence-based care, tailored care, and fewer errors.
Obviously, better diagnostic and treatment outcomes are admirable objectives. However, AI is only as good as the people who program it and the environment in which it runs. If we are not careful, artificial intelligence (AI) could exacerbate many of the worst characteristics of our current healthcare system, rather than improve it. AI systems examine massive volumes of data using deep and machine learning to generate predictions and offer solutions. Large datasets comprising payer claims, electronic health record data, medical imaging, genetic data, laboratory data, prescription data, clinical emails, and patient demographic information can now be created and analyzed cost-effectively because to advances in processing power. This data is completely reliant on AI, and as with everything else in computers, “garbage in, garbage out.” All of our healthcare records perfectly capture a history of arbitrary and unfair inequities in access, treatments, and outcomes across the United States, which is a major source of concern.
Non-whites continue to have worse outcomes for infant mortality, obesity, heart disease, cancer, stroke, HIV/AIDS, and total mortality, according to a 2017 report by the National Academy of Medicine on health care inequalities. Surprisingly, Alaskan Natives have a 60% higher infant death rate than whites. Worse, AIDS mortality among African Americans is on the rise. There are significant spatial disparities in outcomes and death even among whites. By combining patient-generated data from pricey sensors, phones, and social media, biases based on socioeconomic class may be increased.
Also Check: Apple iPhone 14 Release date
The data we’re using to train our AI models could end up perpetuating, if not exacerbating, these persistent inequalities, rather than resolving them. The machines cannot and do not check the accuracy of the data they are supplied. Rather, they presume that the data is completely accurate, of high quality, and reflective of the best possible care and outcomes. As a result, the models developed will be optimized to approximate the current outcomes. Even more difficult to address AI-induced inequities is the fact that the models are primarily “black boxes” built by machines, incomprehensible, and significantly more difficult to audit than our current human health-care delivery processes. Another significant issue is that many practitioners make assumptions and treatment decisions that aren’t clearly captured as structured data. Experienced clinicians acquire intuition that helps them to recognise a sick patient even if the numbers being fed into computer programs make him or her look identical to another less unwell patient. As a result, some patients will be treated differently than others for reasons that are difficult to deduce from electronic health record data. Data does not adequately depict this clinical opinion.
When health systems try to incorporate AI, these issues loom big. When the University of Pittsburgh Medical Center (UPMC) looked at the probability of patients arriving in their emergency department dying from pneumonia, the AI model predicted that mortality would be lower if the patients were over 100 years old or had asthma. While the AI model accurately assessed the underlying data, UPMC’s death rates for these two categories were extremely low. The conclusion that they were at a decreased risk was wrong. Rather, their danger was so great that emergency room staff administered antibiotics to these patients before they were even documented in the computerised medical record, resulting in incorrect time stamps for life-saving medications. This type of study could lead to AI-inspired policies that injure high-risk patients without recognising physician assumptions and their impact on data—in this case, proper scheduling of antibiotic treatment. And this isn’t an isolated case; clinicians make hundreds of similar assumptions every day, across a wide range of illnesses.
We must first commit to finding and correcting bias in datasets before entrusting our care to AI systems and “doctor robots.” In addition, AI systems must be assessed not only for the accuracy of their suggestions, but also for whether they perpetuate or reduce inequities in care and outcomes. Create national test datasets with and without known biases to see how well models are tuned to avoid unethical care and illogical clinical advice. We may take it a step further and use peer review to assess findings and make recommendations for AI system improvements. This is similar to the highly effective approach employed by the National Institutes of Health and journals to evaluate funding proposals and research outcomes. These actions could go a long way toward restoring public confidence in AI, allowing patients to receive the kind of unbiased treatment that human doctors should have been giving all along.